Expand research scope and relevance
Expand the scope and relevance of your research with media sentiment and attention scores. Harnessing 20 years of aggregated data from over 23,000 vetted sources, our datasets cover 300,000 traceable assets, people, and places and underpins hundreds of published research papers.
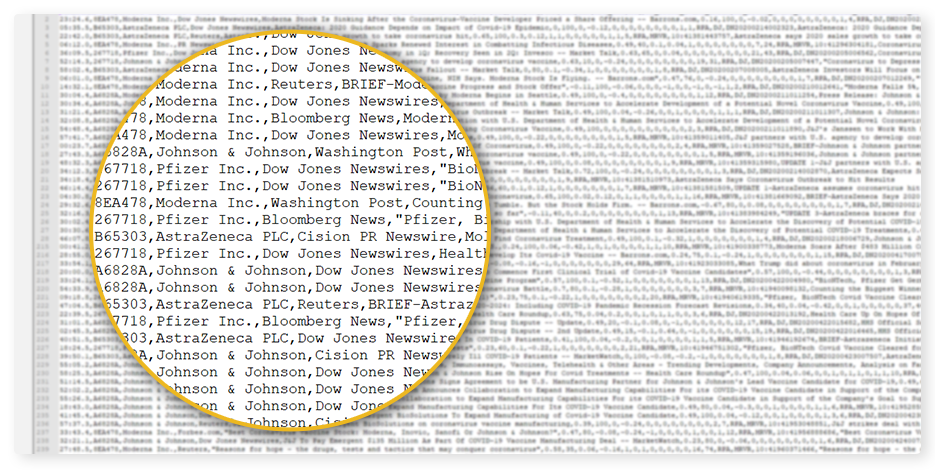
Why researchers choose RavenPack data?
Research models require time-series and quantitative inputs to evaluate statistical correlations, test predictive powers, and validate hypotheses. Yet news, social media posts, emails and other textual data lack structure, so traditional research excludes them. RavenPack makes these untapped pools of data accessible in structured, actionable sets so innovative research teams can exploit them in their models.
Study qualitative signals using quantitative frameworks
RavenPack’s natural language processor scans thousands of online news sources, calculating real-time news sentiment at an entity, event, and story level. In a recent MIT study our sentiment scores were used to develop a model that identified ‘sentiment traders’ and predicted their behavior using Powell’s method and Monte Carlo simulations.
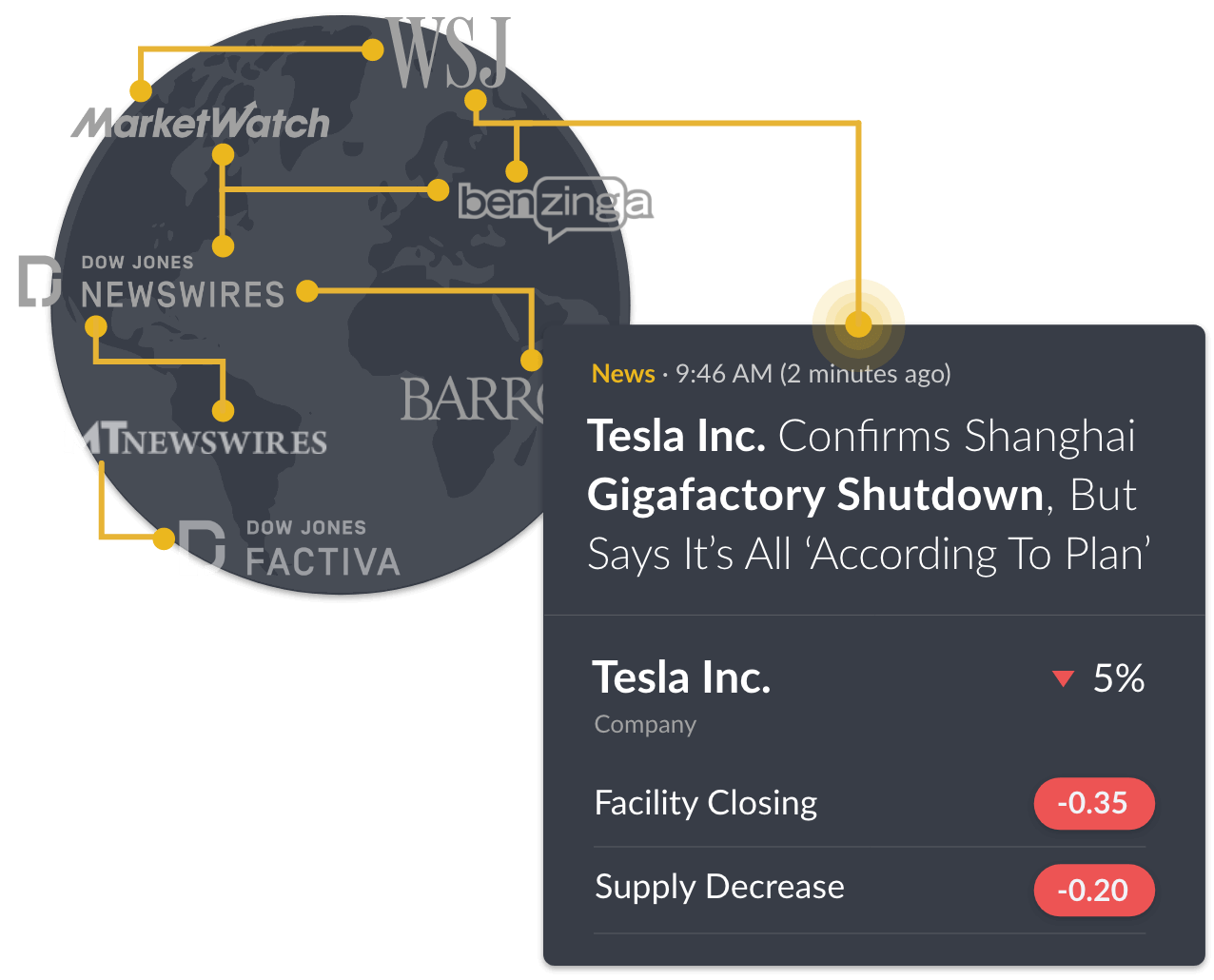
When you need exact timelines and volume of media coverage
Use Media Attention — the volume or share of news about a given subject — as a proxy to track the unfolding of real-world events. A recent study leveraged our Coronavirus monitor’s Panic Index, which models panic based on the share of relevant news, to identify a high degree of correlation between media panic and stock market volatility.
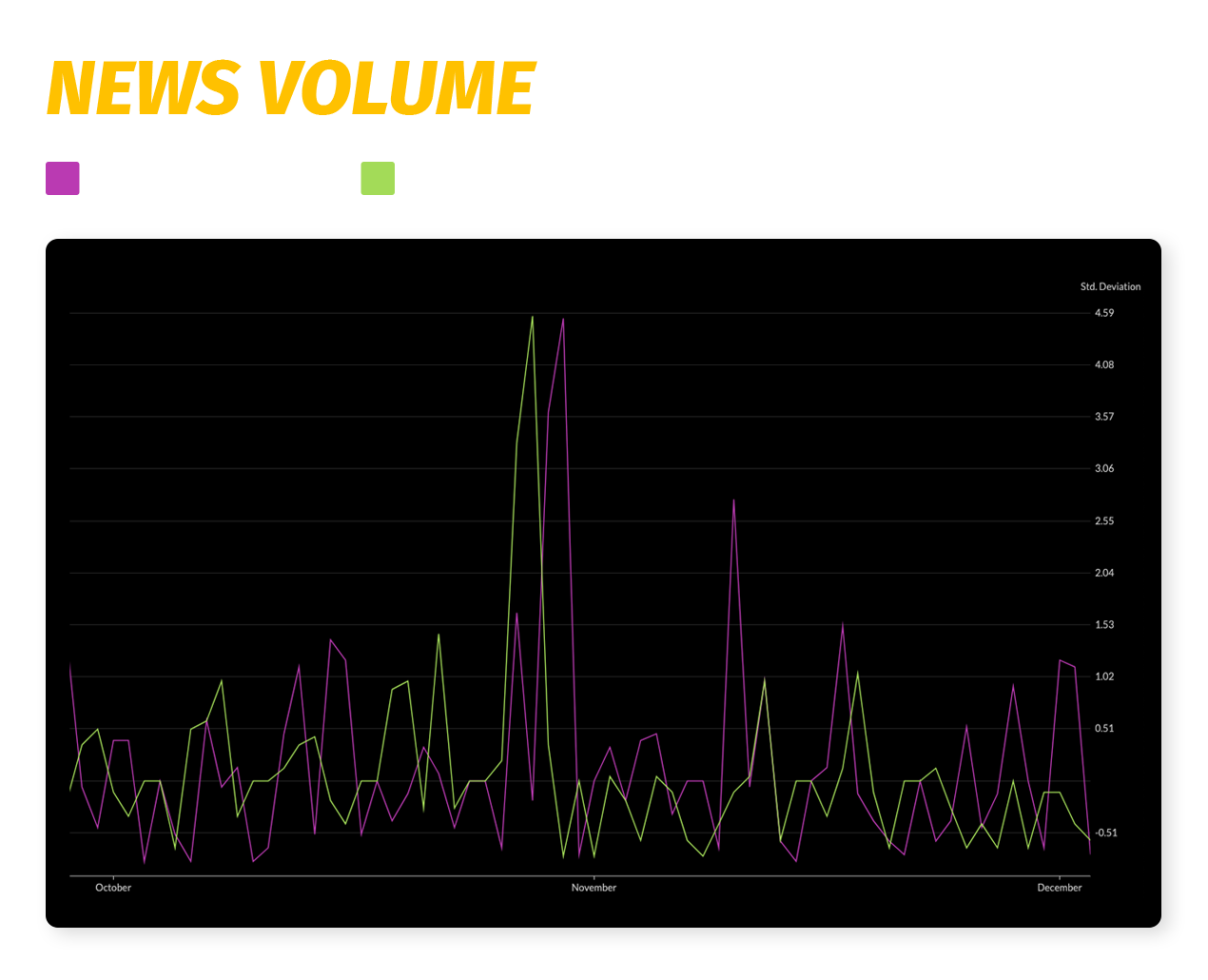
Find statistical significance in large sets of structured data
Researchers can identify bias, patterns, and influences in news and media thanks to the systematic analysis of thousands of sources. A study from the University of Pennsylvania used news analytics to explore whether managers opportunistically exploited heightened media attention around investor conferences to "hype" up the stock price. Another from Boston college used our data to research whether companies led by female CEOs experienced more negative news coverage.
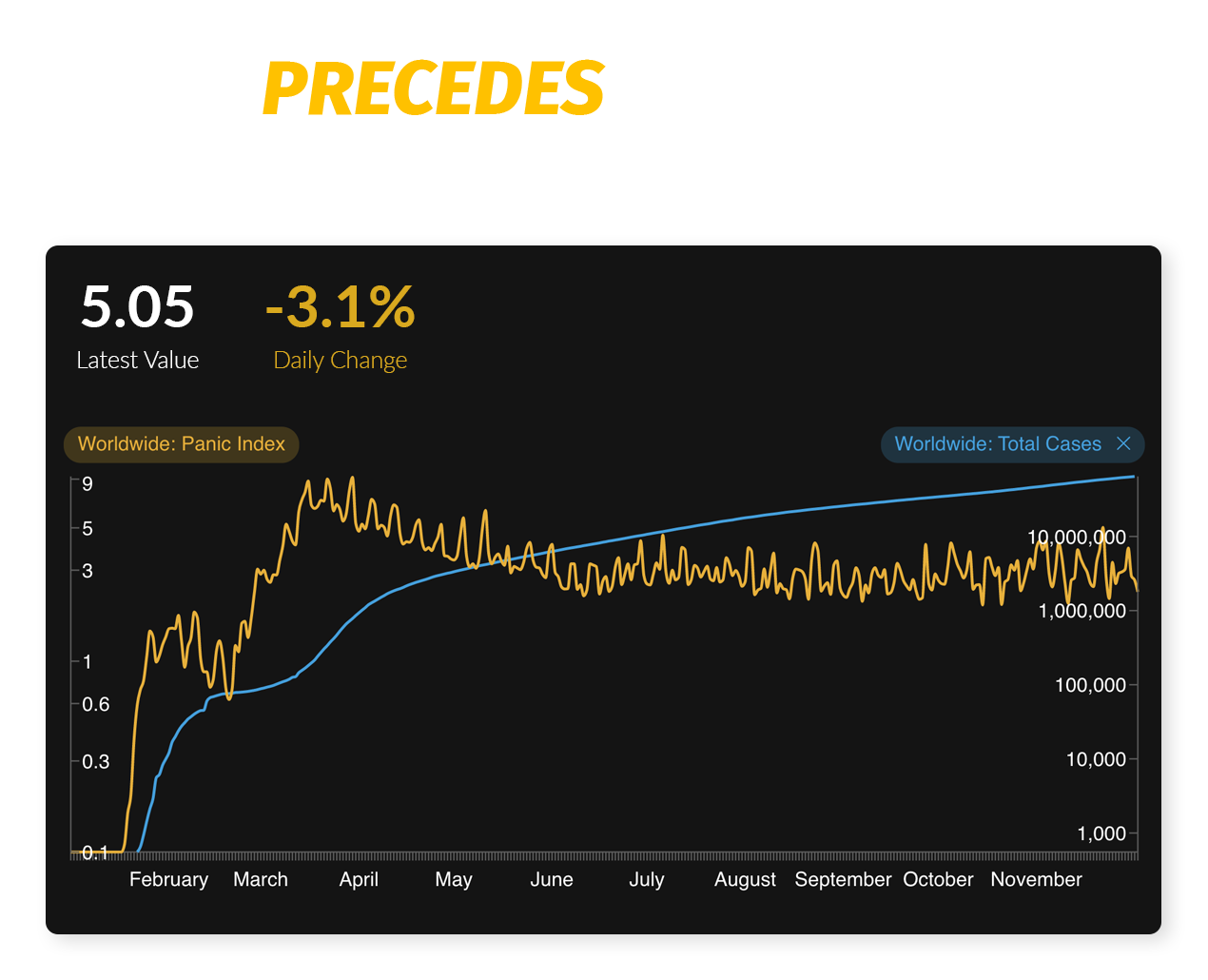
A comprehensive repository of corporate information
The RavenPack dataset includes detailed company information including SEC filings, earnings calendar dates and revisions, insider trades, entity relationships and supply chain data, and all related news to reconstruct, track or analyze business decisions. For instance, a recently published study leveraged RavenPack data to analyze corporate divestments.
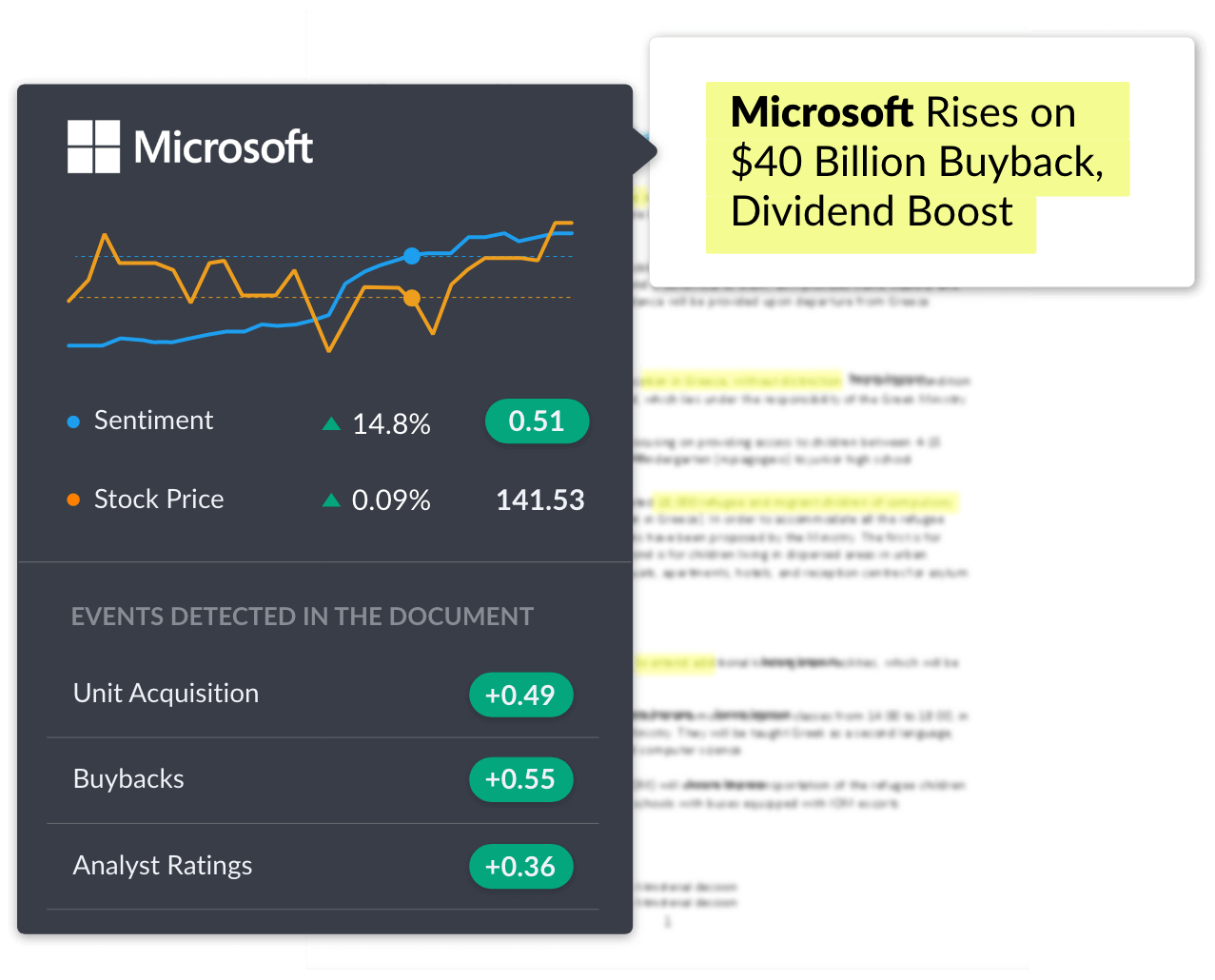
Study qualitative signals using quantitative frameworks
RavenPack’s natural language processor scans thousands of online news sources, calculating real-time news sentiment at an entity, event, and story level. In a recent MIT study our sentiment scores were used to develop a model that identified ‘sentiment traders’ and predicted their behavior using Powell’s method and Monte Carlo simulations.
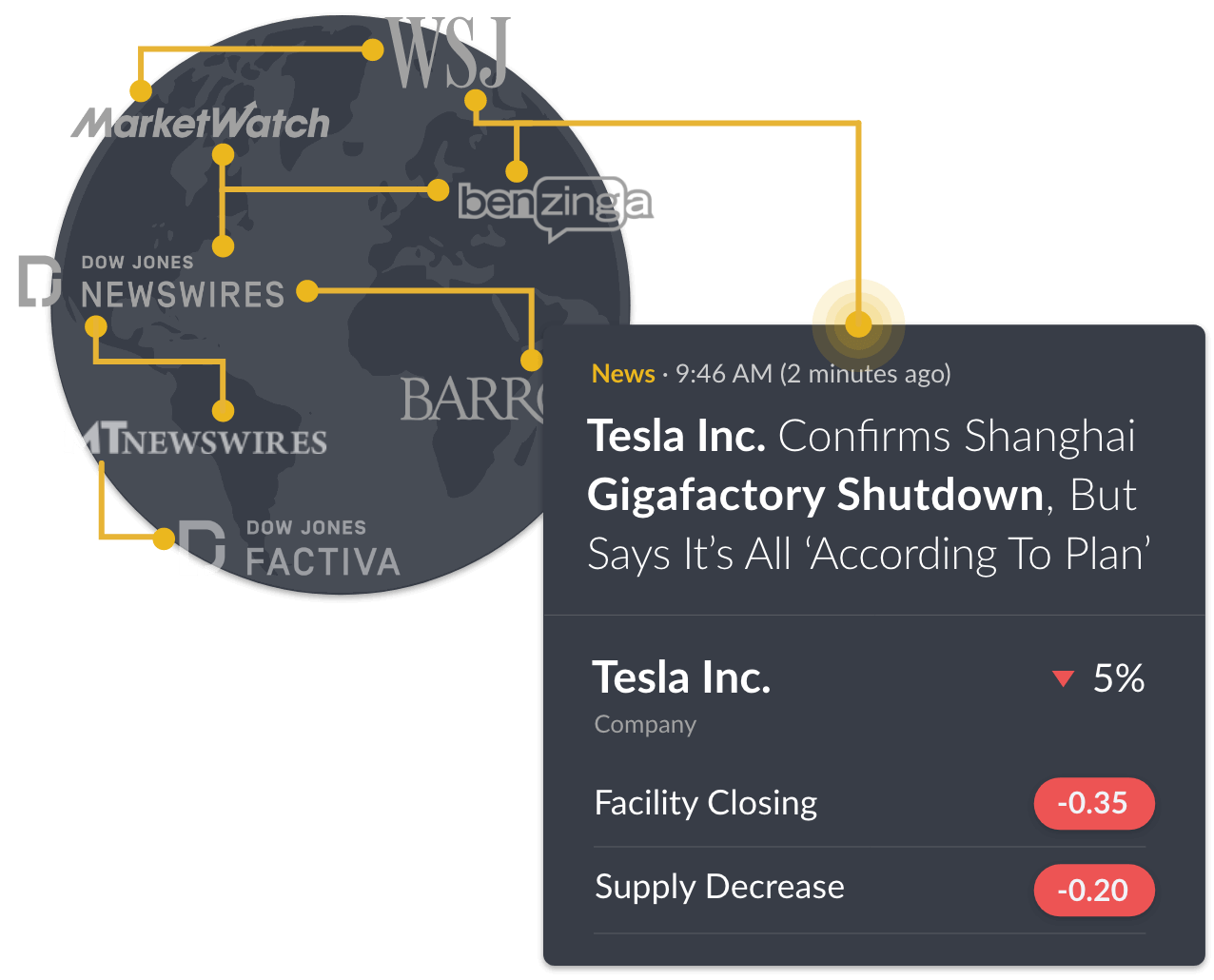
When you need exact timelines and volume of media coverage
Use Media Attention — the volume or share of news about a given subject — as a proxy to track the unfolding of real-world events. A recent study leveraged our Coronavirus monitor’s Panic Index, which models panic based on the share of relevant news, to identify a high degree of correlation between media panic and stock market volatility.
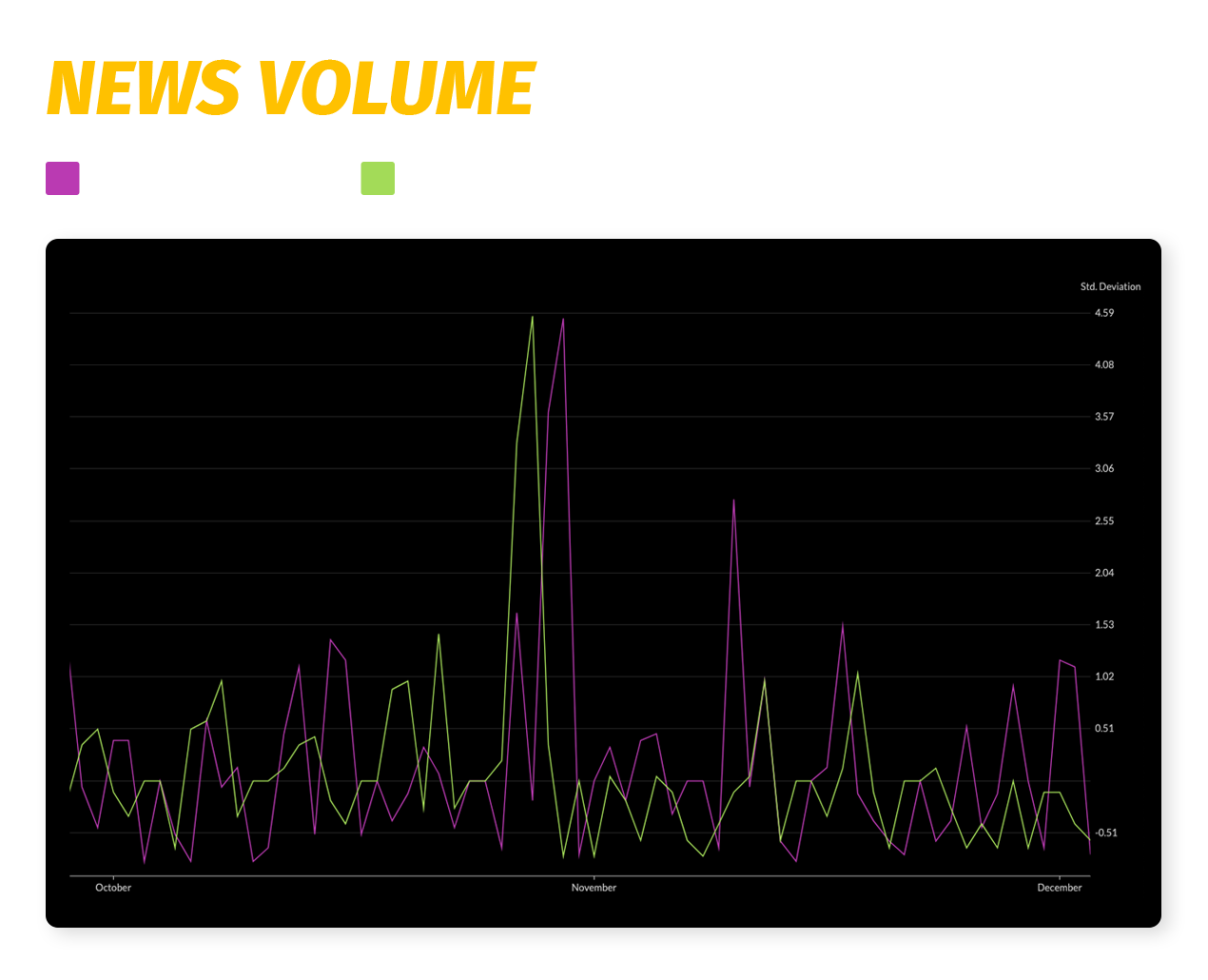
A comprehensive repository of corporate information
The RavenPack dataset includes detailed company information including SEC filings, earnings calendar dates and revisions, insider trades, entity relationships and supply chain data, and all related news to reconstruct, track or analyze business decisions. For instance, a recently published study leveraged RavenPack data to analyze corporate divestments.
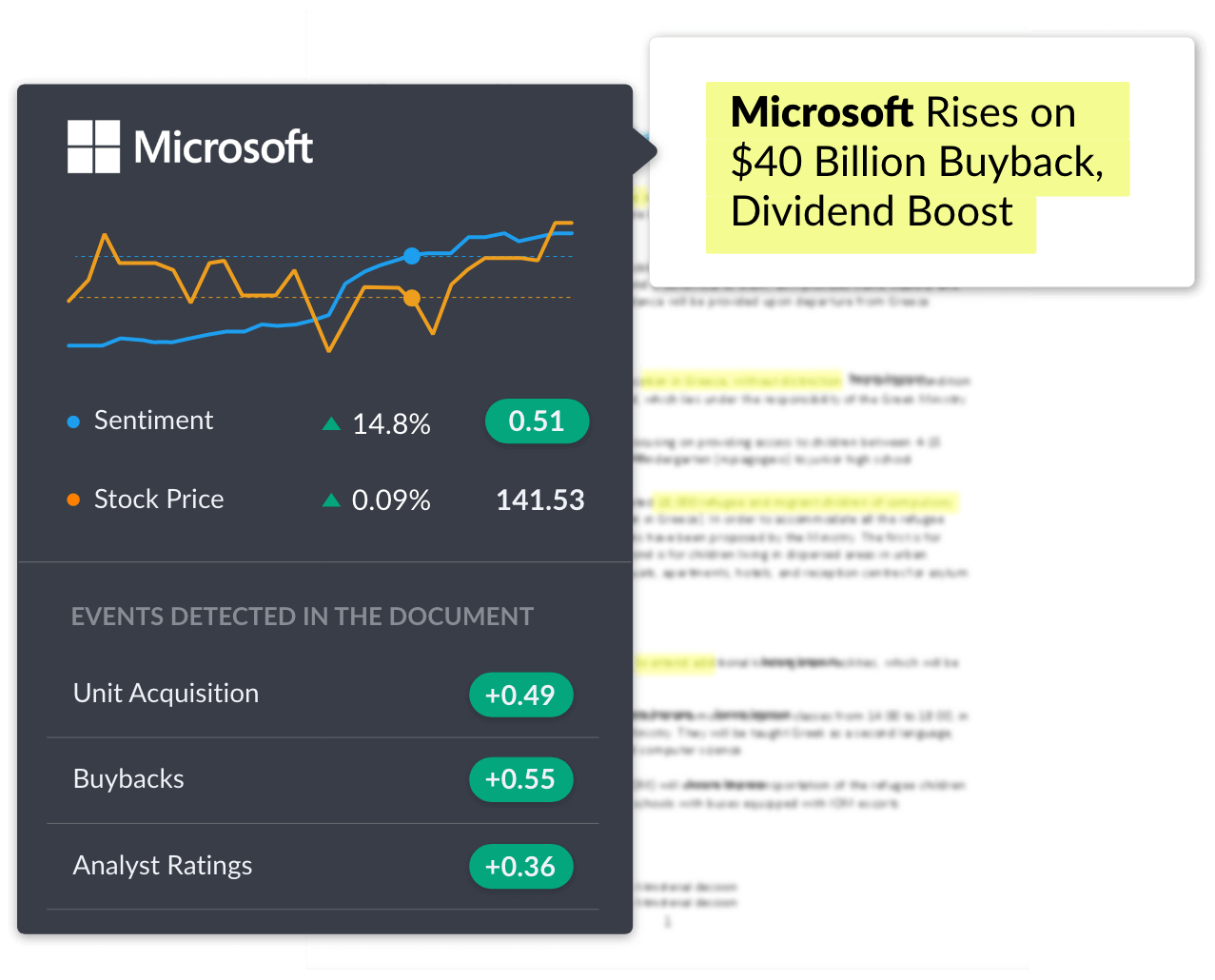
Research applications in:
Finance &
Economics
Public
Health
Political
Sciences
Social
Studies
Environmental, Social and Corporate Governance
Media
Studies
Request a Trial
Fill out the form below and see RavenPack in action.